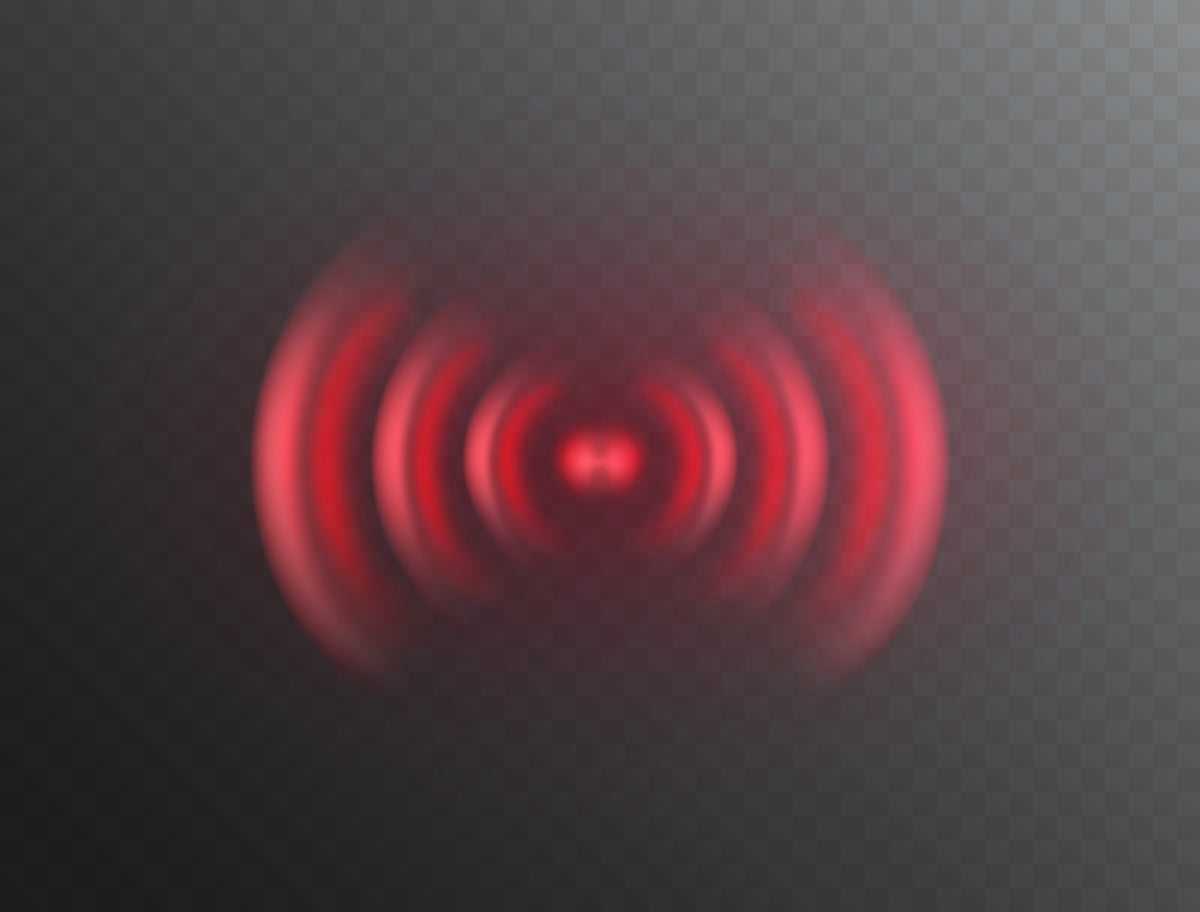
Reducing the Noise from Alerts, Alarms, and Notifications
When technology entered healthcare, so too did alerts. Alerts acted as attention grabbers, reminders, notices of changes in a patient's condition, and warnings about malfunctions or improper functioning. As technology grew more prevalent with the advent of automated dispensing cabinets, computerized provider order entry (CPOE), digital point-of-care monitors and devices, and electronic health records (EHRs), the number of alerts grew exponentially. Clinicians were exposed not only to their own patients' alerts, but to all of the alerts or alarms sounding within a particular unit. The number of per-patient alerts can be astounding. One facility determined that in its critical care unit, "between 150 to 400 physiologic monitoring alarms" sounded per patient, per day.1 This cacophony is compounded by alerts triggered by electronic records.
While alerts from patient monitors, intravenous pumps, beds, door alarms, and sterilization units all create concern, additional problems may arise from the ever-increasing number of alerts generated by CPOE and from the multiple forms of clinical decision support (CDS) now in common use. Numerous alerts and alarms are communicated not only to EHRs throughout the day but also directly to clinicians' personal phones and other devices. While some dispute whether "alert fatigue" is real, given the types and frequency of safety events, technology-related alert fatigue must be given careful consideration.
Alert fatigue is defined as "declining [clinician] responsiveness to a particular type of alert as the [clinician] is repeatedly exposed to that alert over a period of time, [so that they] gradually becom[e] 'fatigued' or desensitized to it".2 Alert fatigue is real. " Alert fatigue caused by one type of alert may also lead to declined responsiveness to other types of . . . alerts." CDS alerts take the form of reminders2 (e.g., renal function in relation to a medication), precautions (e.g., dose range), and warnings (e.g., drug-drug interactions). While these alerts are meant to enhance patient safety, being bombarded with "inconsequential warnings can result in all warnings being ignored."3
The number of alerts received daily, the number of alerts overridden, the safety of patients when anticipated alerts are not received, and the overwhelming nature of these frequent occurrences are the focus of efforts by the current Partnership for Health IT Patient Safety workgroup on Safe Practices to Reduce CPOE Alert Fatigue through Monitoring, Analysis and Optimization. This workgroup is co-chaired by John D. McGreevey III, MD (University of Pennsylvania), Adam Wright, PhD (Vanderbilt), Christina Michalek, BSc Pharm, RPh (ISMP), and Robert Giannini (ECRI).
The multi-stakeholder workgroup anticipates publishing safe practice recommendations following a process comprised of data analysis, an evidence-based literature review, expert debate and consensus building, and study of frontline successes. The workgroup approach to this topic is to delve into monitoring, analysis, and optimization of alerts with the aim of decreasing burden.
First, the workgroup focused on monitoring. To date, monitoring has been done organization by organization, unit by unit, or specialty by specialty; no single unified method has been identified to effectively monitor alerts or to determine their appropriateness. An essential component of alert monitoring is governance (the creation, removal, and optimization of alerts). For effective governance, alerts must first be evaluated. But it becomes readily apparent that consistent measures to evaluate alerts do not exist. What is the correct metric? Is the metric the number of times an alert fires; the number of alerts per order; whether or not an alert is overridden; the number of times an alert is overridden; the reason an alert is overridden, bypassed, or dismissed; the effectiveness of an alert; the total number of interruptive versus noninterruptive alerts; the dwell time; or the number of alerts that are viewed as burdensome?
To inform the process, the workgroup looked to others' successes using a CDS "maturity model."4 The maturity model includes three main legs: content creation; analytics and reporting; and governance and management. Each leg is built on a number of components, including user-centered design, feedback mechanisms, evidence-based guidelines, anomaly detection, data visualization, monitoring and maintenance, and strategic alignment. Identifying ways and tools to implement these components will ultimately help us derive standards for universal application. Developing a way to readily visualize each of these components will create broader learning.
Also included in alert governance is an evaluation of nomenclature. Can we standardize the appearance and the format of alerts for the same occurrence? Can we clarify the reaction to an alert (e.g., remove versus continue, proceed versus cancel, accept versus override)? These alert metrics might be broken down in the larger buckets including descriptive alert metrics—how many alerts per 100 orders or per encounter; performance metrics—what is the specificity, what is the predictive value; or are metrics that reflect clinician interaction and burden a valuable way to frame interventions. Defining and framing these issues, based on focused investigations, will allow for richer collaborative multi-stakeholder analysis.
The working group continues to meet and in the next several months will evaluate the methods of analysis as well as ways to optimize alerts to decrease burden.
Effective alerts are essential to providing safe and effective care while not adding to the proliferation of notifications and alarms. We continue to see the number of alerts, reaction to alerts, and the burden associated with alerts as prominent patient safety issues. Failure to address these issues now will only make future modifications overwhelming. Additional information about this and other Partnership projects can be found at hitsafety.org.
- Sound the alarm: managing physiologic monitoring systems. Jt Comm Perspect Patient Saf. 2011 Dec;11(12):6-11. Also available: https://www.jointcommission.org/assets/1/6/Perspectives_Alarm.pdf.
- Légat L, Van Laere S, Nyssen M, Steurbaut S, Dupont AG, Cornu P. Clinical decision support systems for drug allergy checking: systematic review. J Med Internet Res. 2018 Sep 7;20(9):e258. Also available: http://dx.doi.org/10.2196/jmir.8206. PMID: 30194058.
- Blumenthal KG, Park MA, Macy EM. Redesigning the allergy module of the electronic health record. Ann Allergy Asthma Immunol. 2016 Aug;117(2):126-31. Also available: https://dx.doi.org/10.1016/j.anai.2016.05.017. PMID: 27315742.
- Orenstein EW, Muthu N, Weitkamp AO, Ferro DF, Zeidlhack MD, Slagle J, Shelov E, Tobias MC. Towards a maturity model for clinical decision support operations. Appl Clin Inform. 2019 Oct;10(5):810-9. Also available: http://dx.doi.org/10.1055/s-0039-1697905. PMID: 31667818.